Introduction
The world of Big Data has emerged as a game-changer in the finance and business industry, creating a wealth of opportunities for market researchers and investors. In this digital age, the amount of data generated daily is staggering, with over 2.5 quintillion bytes produced each day. Big Data Analytics plays a critical role in transforming this raw data into actionable insights, driving data-driven decision-making and unlocking new market opportunities.
I. Embracing the Power of Big Data Analytics
As the importance of big data continues to grow, understanding the strategies and tools necessary for harnessing its power becomes increasingly essential. In this article, we will explore the key strategies for successful big data management, the latest advancements in big data analytics techniques and tools, and the importance of big data security and privacy.
II. Tackling Big Data Challenges: The 3 Vs
While big data offers numerous advantages, it also presents its fair share of challenges. Gartner has identified these obstacles as the ‘3 Vs’: volume, variety, and velocity. The sheer scale of data
being generated poses difficulties in storage and processing, while the diverse range of data formats and sources demands efficient integration methods. Furthermore, the rapid pace at which data is produced and updated necessitates real-time processing and analysis capabilities.
III. Adopting a Holistic Approach to Big Data Management
To address these challenges, finance and business professionals must adopt a holistic approach to big data management, encompassing data collection, storage, processing, and security. By doing so, they can harness the power of big data analytics to gain a competitive edge and make more informed decisions in their respective industries.
IV. Exploring Advanced Big Data Analytics Techniques and Tools
In the sections that follow, we will delve deeper into the key strategies for successful big data management, exploring the best practices for data collection and storage, data processing and integration, and data quality and governance. We will also discuss the latest trends and tools in big data analytics, including descriptive, predictive, and prescriptive analytics, as well as real-time analytics and advanced analytics with AI integration.
V. Navigating Big Data Security and Privacy Concerns
Furthermore, we will examine the crucial aspects of big data security and privacy, highlighting the challenges faced in big data environments, best practices for ensuring data security, and the legal and ethical considerations surrounding data protection and privacy. Finally, we will showcase real-world success stories and case studies, demonstrating the transformative impact of big data analytics in various industries and the future outlook for market research and investment.
By exploring these topics in depth, this article aims to provide finance and business professionals with a comprehensive understanding of the strategies, tools, and techniques required for success in the increasingly data-driven world of big data analytics.
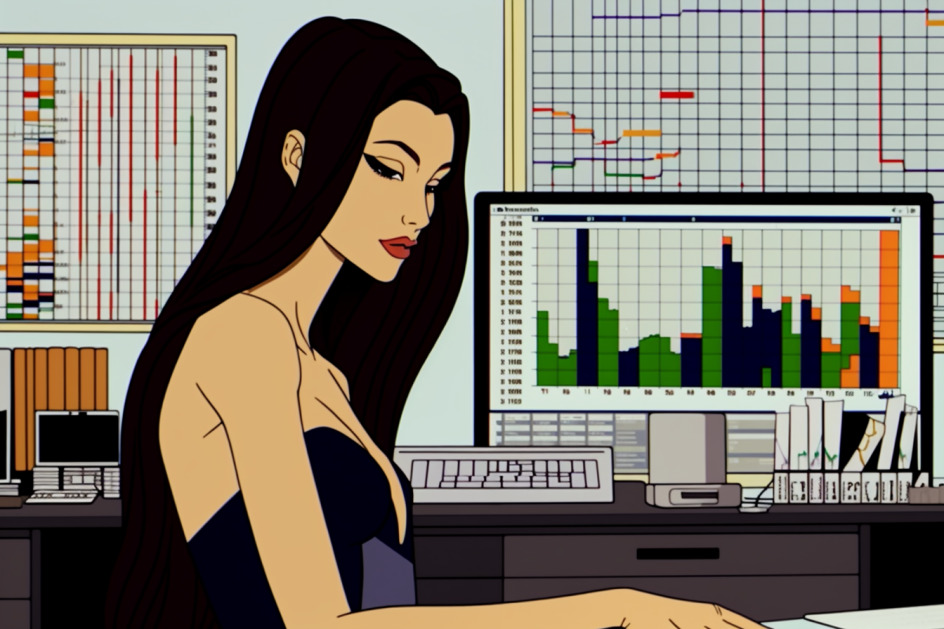
Key Strategies for Successful Big Data Management
Effective Big Data Management is crucial for harnessing the power of big data analytics. In this section, we will explore key strategies for data collection and storage, data processing and integration, and data quality and governance.
A. Data Collection and Storage
1. Selecting the Right Data Sources
Identifying the most relevant and reliable data sources is critical to the success of any big data project. For instance, in the financial industry, data can be sourced from stock exchanges, financial news outlets, corporate financial statements, and social media platforms. Websites like Quandl and Intrinio offer access to a wide range of financial data from various sources, enabling users to make data-driven investment decisions.
2. Data Storage Options: Cloud, On-Premises, and Hybrid
Choosing the right data storage solution is essential for handling the massive volumes of big data. Cloud-based storage options like Amazon S3, Google Cloud Storage, and Microsoft Azure Storage provide scalable and cost-effective solutions for storing and managing data. On-premises storage solutions, such as IBM Storage and Dell EMC, offer more control over data security and compliance. For organizations looking to balance the benefits of both, hybrid storage solutions, like NetApp and HPE, combine on-premises and cloud storage capabilities.
B. Data Processing and Integration
1. ETL Processes and Data Pipelines
Extracting, transforming, and loading (ETL) data from disparate sources into a unified format is critical for effective big data analysis. ETL tools like Talend, Microsoft SQL Server Integration Services (SSIS), and Oracle Data Integrator enable organizations to create and automate data pipelines, ensuring seamless data integration and consistency.
2. Data Integration Tools and Techniques
Various data integration techniques can be employed to combine data from multiple sources, such as data federation, data virtualization, and data replication. Tools like Informatica PowerCenter and IBM InfoSphere Information Server facilitate data integration across different platforms, allowing organizations to gain a comprehensive view of their data landscape.
C. Data Quality and Governance
1. Ensuring Data Accuracy, Consistency, and Completeness
Maintaining high-quality data is crucial for deriving accurate insights from big data analytics. Data quality tools, such as SAS Data Quality and Trillium Software, help organizations identify and resolve data quality issues, including data inconsistencies, inaccuracies, and duplicates.
2. Data Governance Frameworks and Best Practices
Implementing effective data governance frameworks ensures that data is managed, protected, and utilized in compliance with industry standards and regulations. Best practices include creating clear data policies, assigning data ownership, and establishing data lineage. Data governance tools like Collibra and Alation enable organizations to streamline data governance processes and maintain compliance with regulations, such as the General Data Protection Regulation (GDPR) and the California Consumer Privacy Act (CCPA).
Big Data Analytics Techniques and Tools
With the right management strategies in place, organizations can leverage various big data analytics techniques and tools to unlock valuable insights and drive better decision-making.
A. Descriptive Analytics
1. Data Visualization Tools
Descriptive analytics involves summarizing and visualizing historical data to better understand past trends and patterns. Data visualization tools like Tableau, Microsoft Power BI, and Domo enable users to create interactive dashboards and reports, making it easier to interpret and communicate complex data.
2. Real-World Application Examples
For instance, a retail company could use descriptive analytics to analyze past sales data, revealing seasonal trends and popular products. This information could then be used to inform inventory management, marketing campaigns, and pricing strategies.
B. Predictive Analytics
1. Machine Learning and AI Algorithms
Predictive analytics utilizes machine learning and AI algorithms to forecast future outcomes based on historical data. Tools like Vertex Google AI Platform, Amazon SageMaker, and IBM Watson Studio provide a range of machine learning and AI capabilities, enabling users to develop and deploy predictive models with ease.
2. Real-World Application Examples
In the finance industry, predictive analytics can be employed to assess credit risk, forecast stock prices, and identify potential fraud. For example, a bank might use machine learning algorithms to predict the likelihood of loan default based on an applicant’s financial history, allowing for more informed lending decisions.
C. Prescriptive Analytics
1. Optimization and Simulation Techniques
Prescriptive analytics takes data-driven decision-making one step further by recommending specific actions to optimize a desired outcome. This approach often involves the use of optimization and simulation techniques, such as linear programming and Monte Carlo simulation. Tools like Gurobi Optimizer and LINDO Systems offer powerful optimization capabilities for tackling complex business problems.
2. Real-World Application Examples
A supply chain company could use prescriptive analytics to optimize logistics and inventory management, reducing transportation costs and minimizing stockouts. For instance, a transportation routing model could be employed to identify the most efficient delivery routes, taking into account factors like fuel costs, traffic patterns, and delivery deadlines.
D. Emerging Trends in Big Data Analytics
1. Real-Time Analytics
Real-time analytics enables organizations to analyze data as it is generated, providing immediate insights and facilitating more agile decision-making. Technologies like Apache Kafka and Confluent enable the processing of high-velocity data streams, while real-time analytics platforms like Elasticsearch and MemSQL offer fast query capabilities.
2. Advanced Analytics and AI Integration
The integration of advanced analytics techniques and AI capabilities is transforming the way organizations leverage big data. Platforms like SAS Analytics and Alteryx combine a range of analytics functionalities, including data preparation, predictive modeling, and machine learning, enabling users to develop more sophisticated and accurate insights.
Big Data Security and Privacy
As big data becomes increasingly central to business operations, ensuring the security and privacy of this information is of paramount importance.
A. Security Challenges in Big Data Environments
1. Data Breaches and Cyber Threats
Big data environments are often targeted by cybercriminals seeking to exploit valuable information. High-profile data breaches, such as those affecting Equifax and Marriott, highlight the need for robust security measures. Companies must be vigilant in protecting their data assets against ever-evolving cyber threats.
2. Privacy Concerns and Regulatory Compliance
Privacy regulations, such as the General Data Protection Regulation (GDPR) and the California Consumer Privacy Act (CCPA), impose strict requirements on how organizations collect, store, and process personal data. Non-compliance can result in significant fines and reputational damage, making it essential for companies to prioritize data privacy and adhere to all relevant legislation.
B. Security Best Practices
1. Data Encryption and Secure Data Transmission
Encrypting data both at rest and in transit is a critical component of big data security. Encryption technologies like VeraCrypt and GNU Privacy Guard (GPG) help safeguard sensitive data from unauthorized access. Additionally, secure data transmission protocols, such as Secure Sockets Layer (SSL) and Transport Layer Security (TLS), should be employed to protect data during communication between systems.
2. Access Control and User Authentication
Implementing strict access controls and robust user authentication processes helps ensure that only authorized individuals can access sensitive data. Identity and access management (IAM) solutions like Okta and Microsoft Azure Active Directory facilitate the enforcement of access policies and the management of user permissions.
C. Privacy-Preserving Techniques
1. Data Anonymization and Masking
Data anonymization and masking techniques, such as data obfuscation, pseudonymization, and generalization, help protect individual privacy by rendering personal information unidentifiable. Tools like IBM Guardium Data Masking and Delphix Data Masking enable organizations to implement these techniques effectively, ensuring compliance with data protection regulations.
2. Differential Privacy
Differential privacy is a mathematical framework that adds controlled noise to datasets, allowing for useful data analysis while preserving individual privacy. Companies like Apple and Google have integrated differential privacy into their data analysis processes to protect user data without sacrificing analytical utility.
D. Legal and Ethical Considerations
1. Data Protection Regulations (e.g., GDPR, CCPA)
Organizations must be aware of and comply with all relevant data protection regulations, such as the General Data Protection Regulation (GDPR) and the California Consumer Privacy Act (CCPA). Compliance entails implementing appropriate security measures, obtaining user consent for data collection and processing, and ensuring data is stored and processed in accordance with the applicable laws.
2. Ethical Data Usage Guidelines and Frameworks
Beyond legal compliance, organizations should also consider the ethical implications of their big data initiatives. Developing and adhering to ethical data usage guidelines and frameworks can help promote responsible data practices and build trust with customers and stakeholders. Resources like the IEEE’s Ethically Aligned Design and the OECD’s Data-Driven Innovation guidelines can serve as valuable references for establishing ethical standards.
Real-World Success Stories and Case Studies
Understanding how big data analytics has been effectively employed across various industries can help organizations harness its full potential.
A. Improving Market Research with Big Data Analytics
1. Enhanced Customer Segmentation and Targeting
Netflix leverages big data analytics to analyze viewing patterns and preferences, enabling the company to segment its user base and tailor content recommendations accordingly. This targeted approach has helped Netflix achieve higher customer satisfaction and retention rates.
2. Identifying New Market Opportunities
Spotify utilizes big data to discover emerging artists and musical trends, helping the company stay ahead of the curve and capitalize on new market opportunities. By analyzing streaming data and social media metrics, Spotify can identify breakout hits and artists before they become mainstream.
B. Driving Investment Decisions with Big Data
1. Risk Assessment and Portfolio Optimization
Quantitative hedge funds, such as Two Sigma and Renaissance Technologies, employ big data analytics and machine learning algorithms to assess investment risks and optimize their portfolios. By analyzing vast amounts of financial data, these funds can identify patterns and correlations that inform their trading strategies, leading to higher returns.
2. Predicting Market Trends and Patterns
Companies like Predata and Sentifi use big data analytics to predict market trends and patterns by analyzing alternative data sources, such as social media sentiment and news articles. Investors can leverage these insights to make more informed decisions and capitalize on emerging trends.
C. Industry-Specific Applications
1. Retail, Healthcare, and Finance Case Studies
Walmart has successfully used big data analytics to optimize its supply chain management and inventory forecasting. In healthcare, Fitbit employs big data to analyze user activity data, providing personalized health insights and recommendations. In finance, American Express utilizes big data to detect fraudulent transactions and enhance customer experience.
2. Innovative Big Data Solutions and Outcomes
Startups like Palantir and Databricks have developed innovative big data solutions that empower organizations across various industries to make data-driven decisions. These companies have achieved remarkable outcomes, such as improved operational efficiency, enhanced customer experiences, and the discovery of new revenue streams.
D. Key Takeaways and Future Outlook
1. Lessons Learned from Successful Big Data Projects
Successful big data projects highlight the importance of robust data management, effective analytics techniques, and a strong focus on security and privacy. These factors are crucial for harnessing the power of big data and generating meaningful insights that drive growth and innovation.
2. The Future of Big Data Analytics in Market Research and Investment
As big data technologies continue to evolve, the potential for more advanced analytics and AI integration will grow. We predict that real-time analytics, edge computing, and privacy-preserving techniques will become increasingly important in the future, enabling organizations to derive even greater value from their big data initiatives.
Conclusion
If you found our big data analytics report insightful, be sure to explore our other industry reports that dive deep into various sectors and their interplay with big data, analytics, management, and security. These comprehensive reports cover topics such as:
- Consumer Banking Services
- Infrastructure as a Service
- Oil and Gas Industry
- Artificial Intelligence in Industry
- Islamic Finance Market
- Credit Cards and Consumer Debt
- Cyber Insurance Coverage
- Digital and Content Marketing
Each report offers a wealth of knowledge and practical insights to help you stay ahead in your industry, leveraging the latest trends and technologies, including big data and analytics. Enhance your understanding of these sectors and make informed decisions for your business or investment strategies.
In conclusion, the transformative potential of big data analytics in the finance industry is immense, driving innovation and empowering decision-makers. By adopting robust data management strategies, leveraging advanced analytics techniques, and prioritizing security and privacy, organizations can harness the full potential of big data. As we move forward, the continuous evolution of big data technologies will open new horizons, shaping the future of market research and investment.